In today’s digital era, network security is more critical than ever. With the rise of sophisticated cyber threats and attacks, traditional security measures are often falling short. Fortunately, Artificial Intelligence (AI) and Machine Learning (ML) are stepping up to fill the gaps, revolutionizing how we approach network security. This article explores how AI and ML are transforming network security solutions, offering enhanced protection against a wide range of cyber threats.
1. The Evolution of Network Security
Network security has traditionally relied on rule-based systems and signature-based detection methods. These methods involve creating a set of predefined rules or signatures that can identify known threats. While effective for known threats, these systems struggle with new, unknown threats and sophisticated attacks.
AI and ML are shifting the paradigm by introducing adaptive, intelligent systems capable of learning from data and improving over time. This evolution is critical as cyber threats become increasingly complex and adaptive.
2. AI and ML in Threat Detection
One of the most significant contributions of AI and ML to network security is their ability to enhance threat detection. Traditional methods often rely on static patterns and known signatures, which can be bypassed by advanced threats. AI and ML, on the other hand, use dynamic analysis and pattern recognition to identify anomalies and potential threats.
Anomaly Detection: AI and ML algorithms can analyze vast amounts of network traffic data in real-time to detect unusual behavior. For instance, if a network normally experiences a certain amount of traffic and suddenly there is a significant spike, AI-driven systems can flag this as suspicious. These systems learn from historical data to identify patterns and deviations that may indicate a security breach.
Behavioral Analysis: Instead of relying solely on known threat signatures, AI and ML can model normal user behavior and detect deviations from this baseline. For example, if an employee’s account suddenly starts accessing files it typically does not, or logs in at unusual hours, AI systems can detect these anomalies and trigger alerts.
3. Automating Incident Response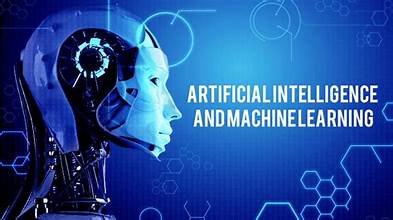
The rapid identification of threats is only part of the solution. Effective network security also requires prompt and accurate incident response. AI and ML are streamlining this process by automating many aspects of threat response.
Automated Responses: AI systems can be programmed to take predefined actions when certain threats are detected. For instance, if a potential malware infection is identified, an AI system can automatically isolate the affected segment of the network, preventing further spread of the malware.
Incident Triage: AI and ML can prioritize security incidents based on their severity and potential impact. This helps security teams focus on the most critical threats first, improving overall response efficiency and reducing the risk of damage.
4. Enhancing Threat Intelligence
Threat intelligence involves gathering, analyzing, and sharing information about potential and existing cyber threats. AI and ML significantly enhance threat intelligence by providing deeper insights and more accurate predictions.
Predictive Analytics: By analyzing historical attack data and current trends, AI can predict future threats and vulnerabilities. This proactive approach allows organizations to strengthen their defenses before an attack occurs.
Intelligence Fusion: AI systems can aggregate data from various sources, such as threat databases, security reports, and real-time network traffic. This fusion of information provides a comprehensive view of the threat landscape, helping organizations stay ahead of emerging threats.
5. Challenges and Considerations
While AI and ML offer substantial benefits for network security, they are not without challenges. Here are some key considerations:
False Positives: AI systems can sometimes generate false positives, where legitimate activities are flagged as threats. Continuous tuning and training of AI models are required to minimize these occurrences.
Data Privacy: The use of AI and ML involves analyzing large volumes of network data, raising concerns about data privacy. Organizations must ensure that their AI-driven security solutions comply with privacy regulations and protect sensitive information.
Complexity and Costs: Implementing AI and ML solutions can be complex and costly. Organizations need to evaluate their specific needs and resources to determine the best approach for integrating these technologies into their security infrastructure.
6. The Future of AI and ML in Network Security
The role of AI and ML in network security is expected to grow as technology advances. Future developments may include:
Enhanced Machine Learning Models: Continued improvement in ML algorithms will lead to more accurate threat detection and response capabilities.
Integration with Other Technologies: AI and ML will increasingly integrate with other emerging technologies, such as blockchain and quantum computing, to provide more robust security solutions.
Adaptive Security Architectures: Future security systems will become more adaptive, learning from evolving threats and continuously improving their defenses.
Conclusion
AI and Machine Learning are transforming network security by providing more advanced, adaptive, and efficient solutions for detecting and responding to threats. These technologies offer significant advantages over traditional security methods, including improved threat detection, automated incident response, and enhanced threat intelligence. As AI and ML continue to evolve, they will play an increasingly critical role in safeguarding networks against the ever-growing range of cyber threats.